Product analytics
Product recommendation engines
Improve cross-selling and upselling, with personalised product offers for every customer
What is a product recommendation engine?
Through algorithms and machine learning, product recommendation engines analyse past behaviour, customer preferences, and historical transaction data to generate the most relevant and personalised product suggestions for customers.
These engines play a crucial role in improving engagement rates, increasing basket size and driving revenue for many businesses.
Recommendation engines can constantly improve over time, and enable you to personalise customer product offers before they have declared interests of left a traceable online footprint.
Relevant offer targeting
Reduce abandoned carts
Maximise customer spend
Increase customer satisfaction
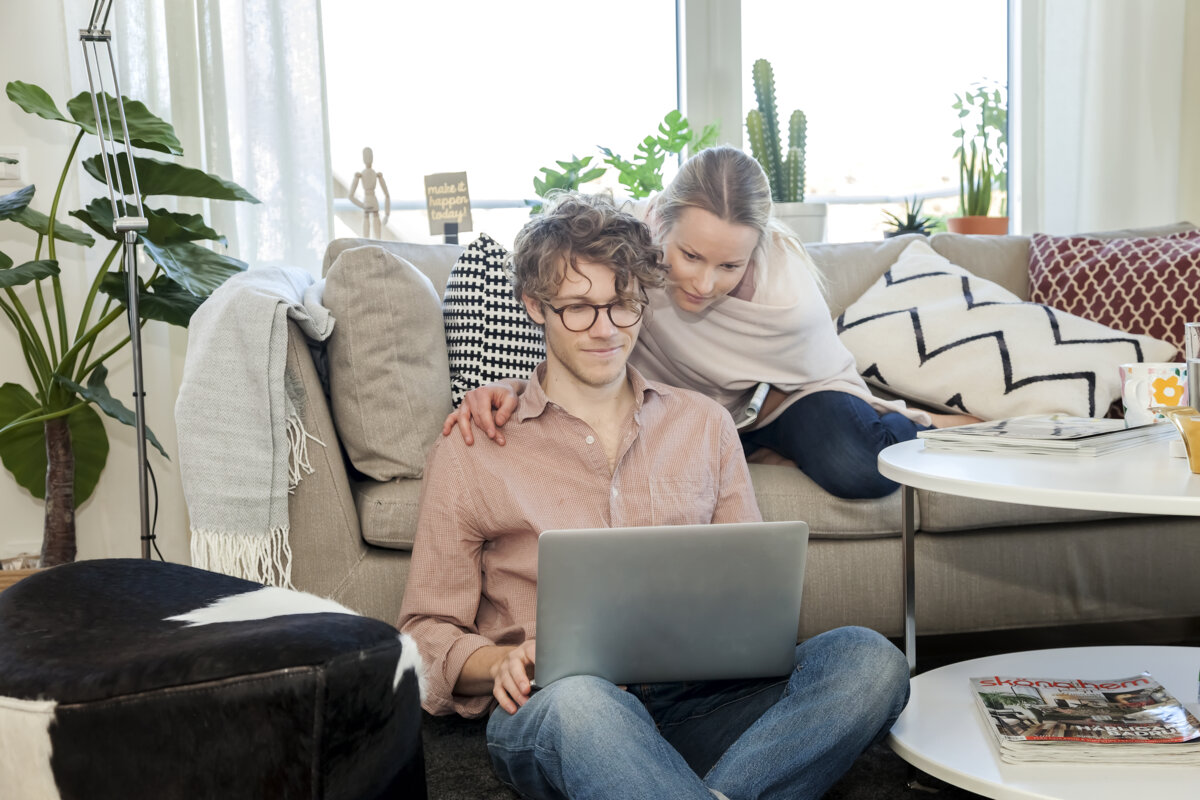
Frequently asked questions
How do product recommendation engines work?
Recommendation engines work by analysing user data such as browsing history, past purchases and demographic information to predict what products a user might be interested in.
Algorithms such as content filtering, collaborative filtering or a combination of them both can be used to make these predictions.
What filtering methods does a recommendation engine use?
Collaborative filtering relies on user behaviour and preferences. It identifies users who have similar preferences and recommends items or content that those similar users have purchased or interacted with.
Content-based filtering recommends products based on the attributes and characteristics of the products themselves. It looks at features or themes of the items and suggests similar or related items based on those attributes.
Some engines can use a hybrid of both techniques to provide more accurate or diverse product recommendations.
How do recommendation engines handle privacy & data security concerns?
Recommendation engines should implement data anonymisation techniques, encryption, and access controls to protect user data and address privacy concerns.
How can businesses measure the success of their product recommendation engines?
Some of the success metrics for product recommendation engines include an increase in click-through rates, conversion rates, customer satisfaction and overall revenue as well as a reduction in basket abandonment.
Do you need to grow customer value faster?
Improving marketing with relevant, personalised recommendations in your communications will drive order values and customer loyalty.
If you’d like to know more and discuss your specific requirements, just get in touch here: