People analytics
Value modelling
How IKEA achieved a 9:1 ROI on targeted, personalised email communications
RFV modelling delivered 9:1 ROI from loyalty scheme customers
Maximising customer engagement and value requires constant communication, and IKEA are experts in this field.
RFV modelling was applied to the base to enable a segmented offer strategy deployed through email and MMS.
The RFV model was then overlayed with a recommendation engine to determine the optimum offer for each value based customer segment.
Taking all costs into account, the campaign achieved a ROI of 9:1
You can read the full case study here, or understand more about RFV modelling below.
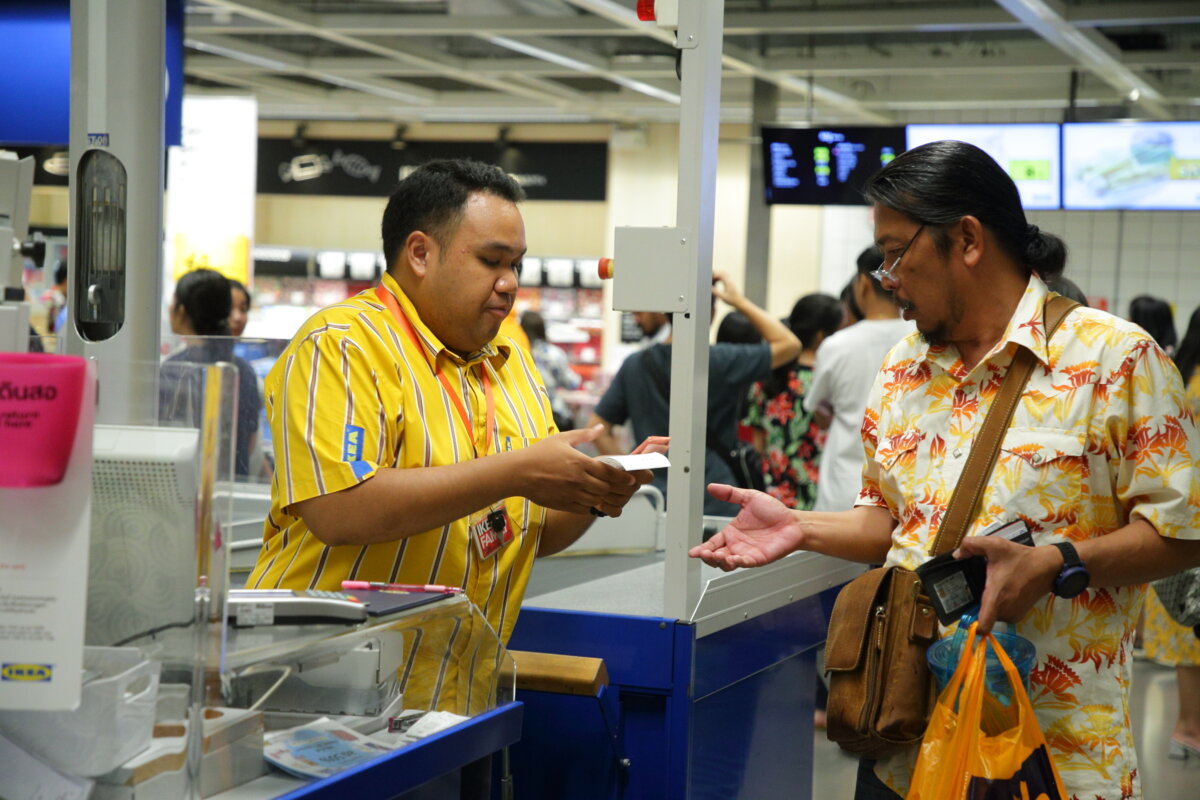
Target customers and personalise communications based on value and engagement
Value modelling segments and analyses customers based on their previous engagement and interaction with a business.
RFV data allows a score to be allocated to every customer and from this businesses can take action to target the value potential in their customer base, maintain growth and stem decline.
Value modelling also optimises marketing efforts, increasing customer retention and maximising ROI, as it focuses resource on the customer segments that are most important to your growth.
Recency of purchase
Frequency of purchase
Value of purchase
Scoring and segmenting customers
Targeting growth potential
Maintaining market share growth
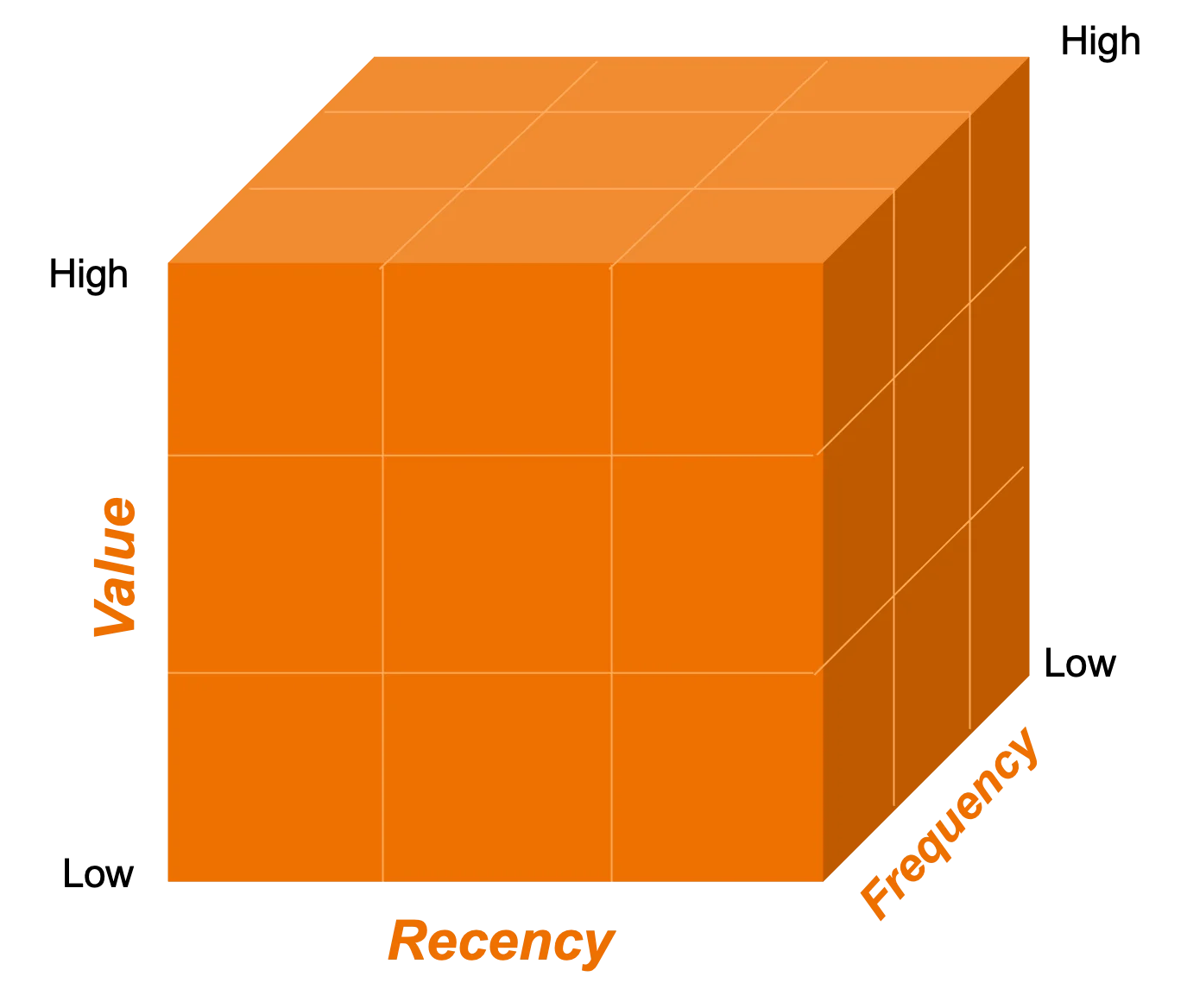
Book a demo to grow your customer value
If you need to unlock value or add external value to your data, to grow market share or customer spend, then we can help.
Our analysts will chat with you about your objectives and identify the best approach for your business. Just choose a time that suits you.
Frequently asked questions
What does RFV stand for?
- Recency (R) refers to how recently a customer has made a purchase or engaged with your brand. It helps determine how active customers are with your business.
- Frequency (F) quantifies how often a customer makes a purchase. It can identify whether a customer is a frequent or more occassional buyer.
- Value (V) refers to the total amount of money a customer has spent with a business over a set time period. It indicates the level of profitability a customer brings to the business.
Why is RFV modelling important for business?
RFV modelling is important as it helps businesses identify and segment customers based on their behaviour.
It allows for targeted marketing strategies, personalised recommendations & promotions, and improved customer experience which results in higher levels of retention.
How is the RFV score calculated for customers?
An RFV score is calculated by assigning a numberical value to recency, frequency and monetary metrics, usually on a scale from 1-5 or 1-10 for each. These are then totalled to create an overall RFV score.
Generally higher scores indicate more valuable customers – those that are highly engaged and spend more.
What are the challenges with RFV modelling?
Some challenges include defining appropriate score ranges, handling outliers, ensuring the quality of the data, and understanding that RFV alone may not capture all aspects of customer behaviour or preferences.
What data sources are typically used in RFV modelling?
Some of the data sources we would expect to use when creating an RFV model include transaction data, past purchases data and customer contact data (for recency).
Can RFV modelling be applied to both online and offline businesses?
Yes, RFV modelling can be applied to all types of businesses as long as the right data sources are available.
How can RFV modelling help with customer retention?
Rather than implementing a “one-size fits all” approach to communications and recommendations, having an RFV model allows for a more targeted approach.
For example, once businesses have identified high-value and potentially lapsed customers, they can tailor their strategies to either focus on reward & retention or reactivation.
Do you need to grow customer value faster?
RFV modelling enables you to target the right customers in your marketing, who offer the best value growth potential, with specific messages to drive purchase.
If you’d like to know more and discuss your specific requirements, just get in touch here: